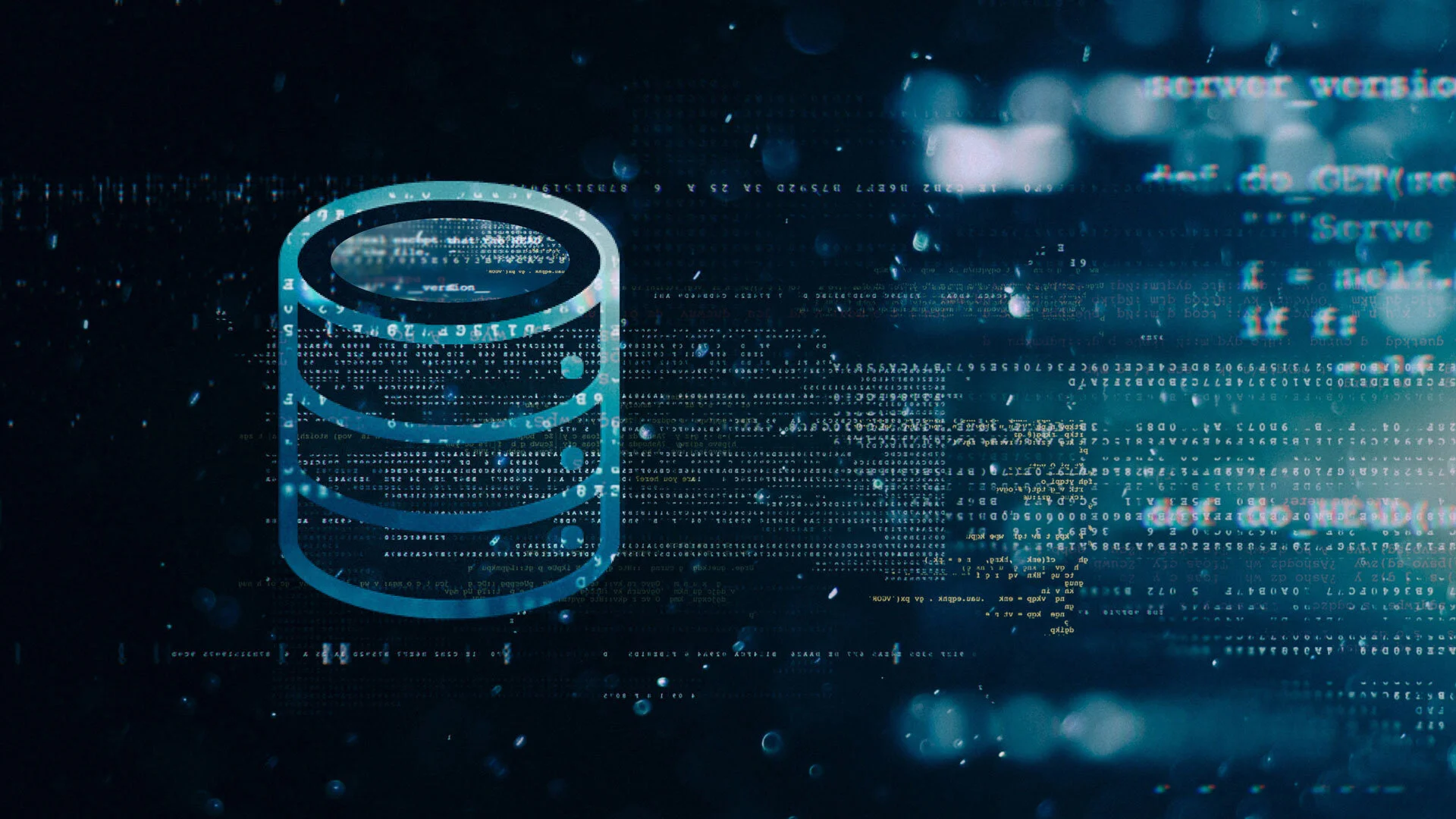
What is data used for?
Gaining an advantage as a data-driven organization
Examples of General Use Cases
Analytics: backward-looking
AI & Machine Learning: forward-looking
Cost prediction
Forecasting, e.g. inventory demand
Pricing; price discrimination based on customer profiles
HR: use past data to predict whom to hire, whom to let go, whom to promote
Customizing distribution, including advertising
Customizing products and services
Analyze reviews and customer feedback
Build new businesses (in other industries), build partnerships
Sell your data (data license agreement with other firm, join marketplace, sell to data agregator) or provide data-driven services
Examples of Specific Use Cases
You can find many examples of data science applications within Kaggle Competitions
Microsoft: developing a gesture recognizer for Microsoft Kinect which was originally meant to be a game controller for Xbox and was then considered for non-gaming applications such as robotics and health care.
Merck: predicting molecular activity for medicines and identifying molecules that are highly active toward their intended targets but not toward other targets that might cause side effects.
GE: making flights more efficient and avoiding costly delays by providing pilots with real-time information on gate conflicts, operational challenges and air traffic management (prize money 250 000$!)
Walmart: forecasting sales for product categories in their store based on sales history, macroeconomic variables, events and holidays, etc.
Brynjolfsson and McAfee (HBR, 2012) who also wrote The Second Machine Age provide examples of data-driven decision making:
Sears Holding collected huge amounts of customer, product and promotion data from its brands. It was clear that it would be valuable to combine these data to personalize offers. Sears reduced the time needed to generate personalized recommendations from 8 to less than 1 week by setting up a Hadoop cluster (named after the toy elephant of the founder), rather than accessing fragmented data warehouses maintained by different brands.
Once shopping moved online, online retailers like Amazon could track not only what customers bought, but also what else they looked at; how they navigated through the site; how much they were influenced by promotions, reviews, and page layouts; and similarities across individuals and groups. They developed algorithms to predict what individual customers would like to buy next—algorithms that performed better every time the customer responded to or ignored a recommendation. Traditional retailers simply couldn’t access this kind of information, let alone act on it in a timely manner.
The Importance of Leadership, Culture and Talent
Ask the right questions.
Set clear goals, define success and ask the right questions: “Computers are useless. They can only give you answers.” (Pablo Picasso)
Address a problem.
More or better data is not enough. Focus on data which address a problem. Choose a practical approach.
Make data-driven decisions.
Do not justify and legitimise decisions by finding the numbers. Important decisions are often made with the “HiPPO” - approach (the highest-paid person’s opinion or intuition) and then reports are spiced up with data.
Maximise cross-functional cooperation.
Bring together people who understand the problems with the right data and with the people who have the problem-solving skills to exploit the data.
Focus on business wins.
Data Analytics must yield clear business wins quickly and iteratively.
Hire great people.
As data become cheaper, the complements to data become more valuable. Some of the most crucial of these are data scientists and other professionals skilled at working with large quantities of information. The best data scientists are also comfortable speaking the language of business and helping leaders reformulate their challenges in ways that big data can tackle. People with these skills are in high demand.
Typical Challenges
“We think we have valuable data but don’t know what to do with it." -> see more on what data is used for above in Data-Driven Advantage
“Data is in many places. It is fragmented and needs to be integrated.” -> see more on structuring your data in Data Architecture
“We cannot communicate our business needs to our data scientists.” -> hire the right people and maximise cross-functional collaboration (see above) and improve data access and structure (see Data Architecture)
“Our customers are asking for explanations.”-> see more on transparency, explicability, and privacy in Policy & Ethics
Other?