What is right?
Privacy, Cybersecurity, Transparency, Unbiasedness, Explicability
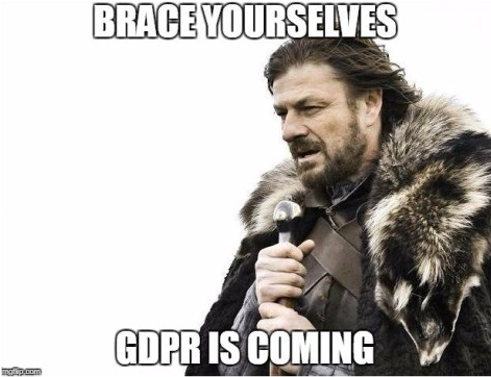
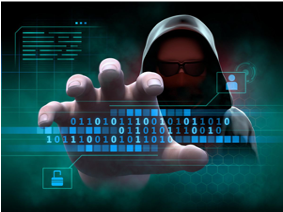
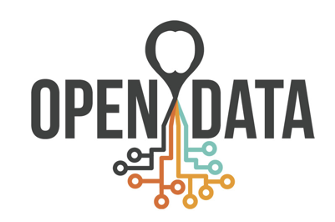
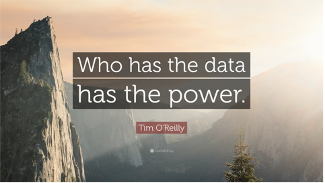
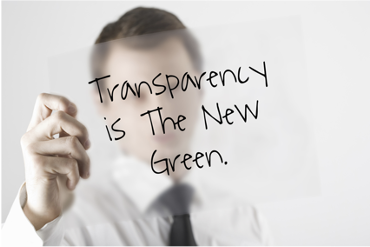
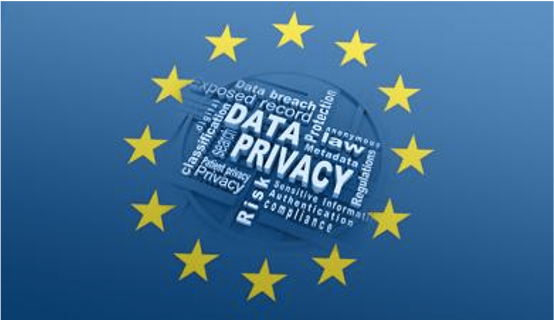
General Data Protection Regulation
EU regulation since April 2016, enforceable since May 2018
Applies to all organisations which collect data on EU citizens (including firms outside the EU)
Fines for violations of up to €20 million or 4% of global revenue (whichever is higher), plus compensation for damages.
Key Principles
Key Principles include:
Data minimisation: firms should collect and store only as much data as necessary for specified purposes
Accuracy: Personal data must be kept accurate and up to date
Transparency: with respect to the provision of information (Art. 13-14), e.g. plainly-worded and unambiguous, to communications of customers’ rights (Art. 15-22) and to data breaches (Art. 34)
Right of access: if a customer requests it, a firm must be able to provide information on categories of data it processes as well as a copy of the customers’ actual personal data (Art. 15)
Right to be forgotten: if a customer requests it, a firm must (be able to) delete his or her personal data within 30 days (Art. 17)
Personell: all organisations should have someone tasked with monitoring GDPR compliance (firms that meet certain criteria, e.g. large scale regular monitoring of data subjects, should hire a data protection officer.
Consent: Data can only be processed with informed consent for each purpose (Art. 4 and 7)
Privacy by Design: Data protection should be designed into the development of business processes for products and services, throughout the whole processing lifecycle (Art. 25)
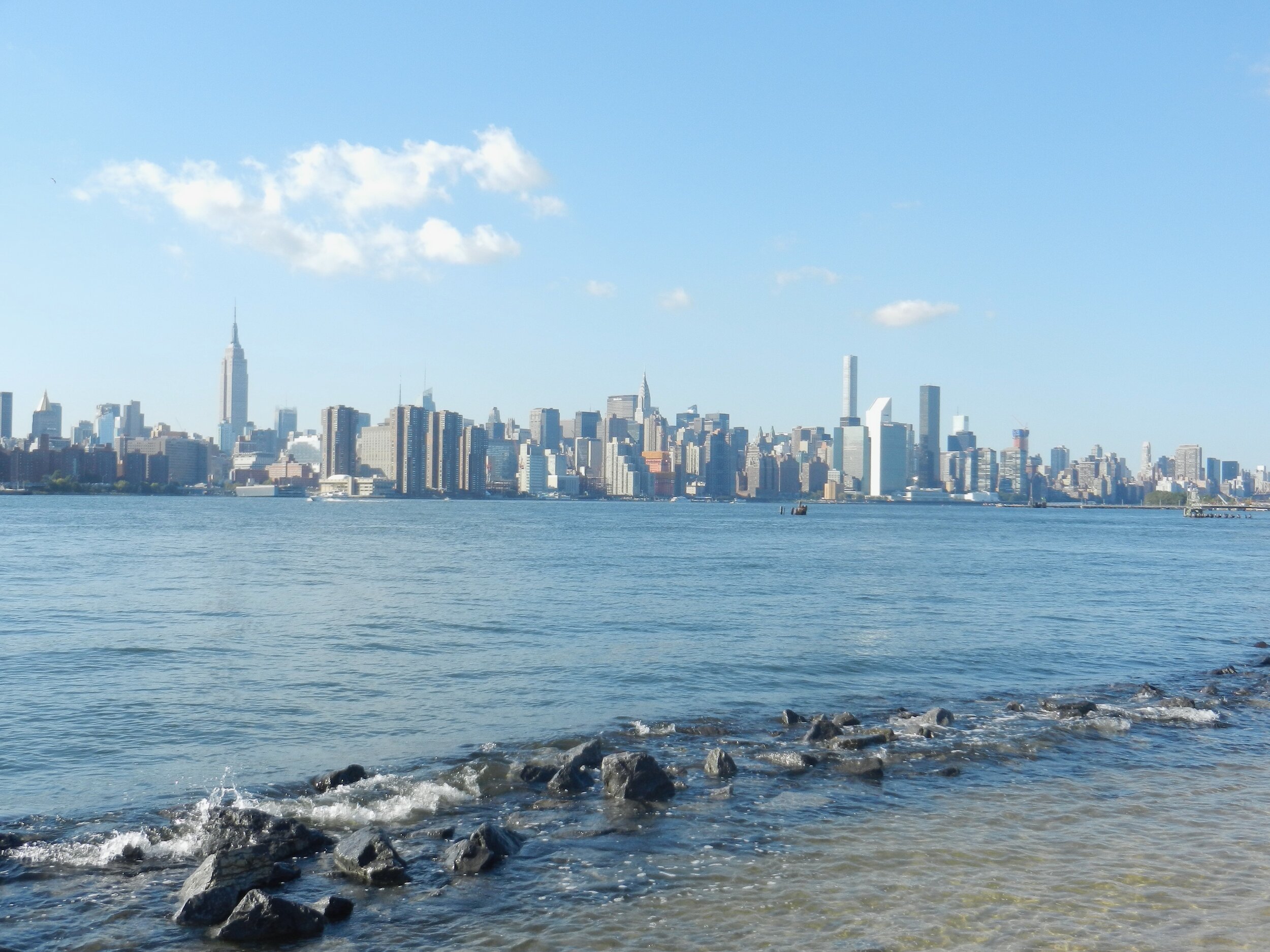
Privacy Policy in the United States
Nation
The United States does not have a comprehensive national data privacy policy. The Federal Trade Commission (FTC) has broad jurisdiction to prevent unfair or “deceptive trade practices.”
Vertically-focussed privacy laws include HIPAA (1996, healthcare, protects patient data), GLBA (financial personal data), COPPA (personal data of children)
The Privacy Shield Framework was designed to provide companies with a mechanism to comply with data protection requirements when transferring personal data from the EU to the United States. In July 2016, the European Commission deemed the Privacy Shield adequate under EU law. However, in July 2020, the European Court of Justice declared this decision invalid (Schrems II), with significant implications for the use of US cloud services since customers are now responsible to ensure compliance with EU law. While joining the Privacy Shield is voluntary, the commitment to its principles is (still) enforcable under US law by the FTC.
States
States’ individual laws: only California and New York have passed laws that are similar to GDPR in their intent and broad application:
California Consumer Privacy Act (CCPA):
Signed into law in June 2018, enforceable since January 2020
Gives consumers the right to access, the right to delete, the right to opt-out of processing at any time
CCPA does not require explicit consent. A privacy notice on the website providing consumers with a right to opt out is sufficient.
New York’s SHIELD Act:
SHIELD stands for Stop Hacks and Improve Electronic Data Security
Enforceable since March 2020
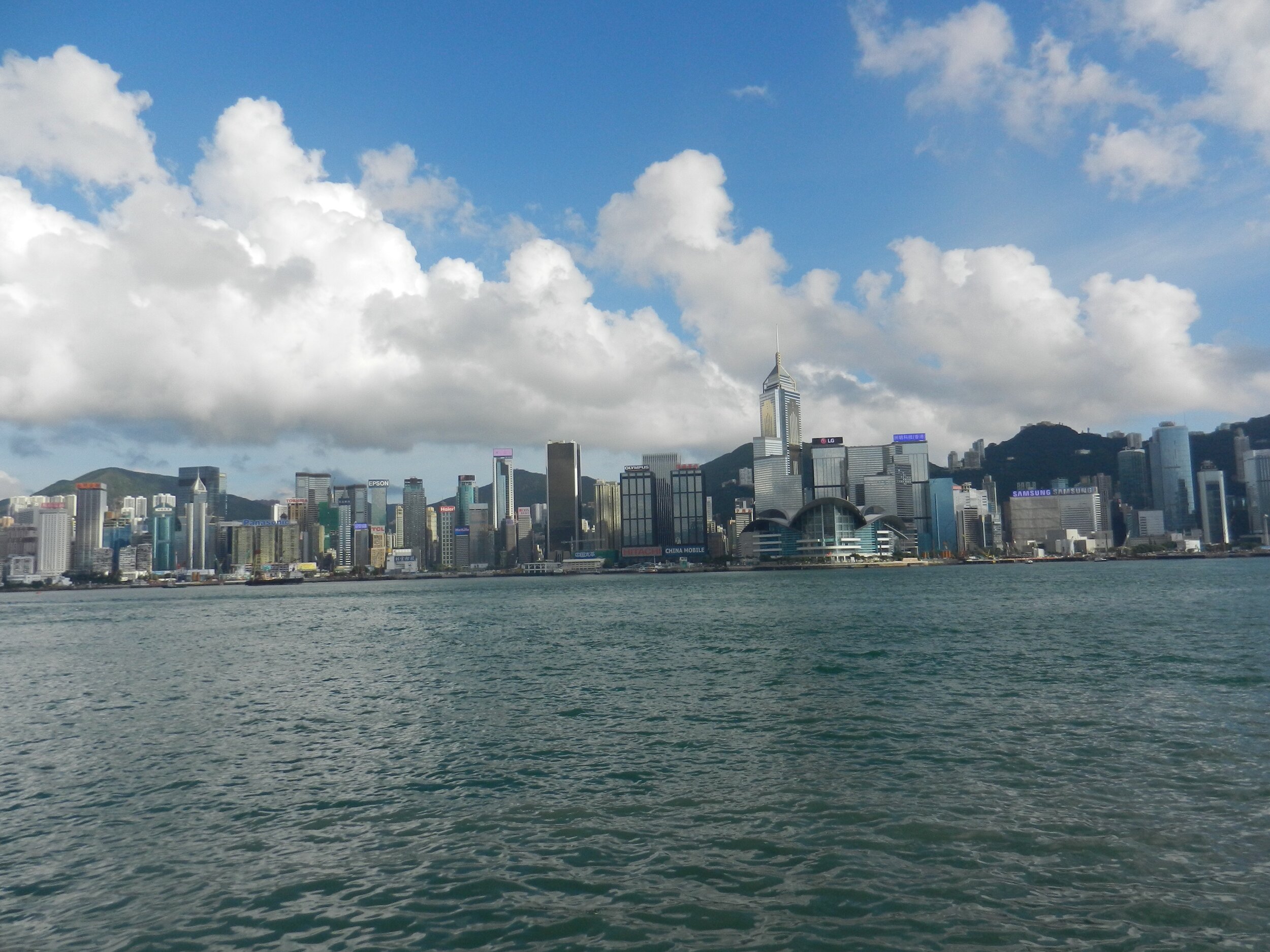
Privacy Policy in China
Despite Chinese people being less sensitive as people in the United States or Europe when it comes to personal information and privacy, China has recently implemented a Cybersecurity Law (2017) and issued a national standard on personal information (2018).
The Cybersecurity law took effect in June 2017 and banned online service providers from collecting and selling users’ personal information without user consent. It prohibits network operators from gathering data not relevant to their services, and from sharing data without consent.
In March 2018, China issued a national standard which is not legally binding but referred to by the government when conducting reviews and approvals, creating strong incentives for compliance. It covers collection, storage, use, sharing, transfer, and disclosure of personal information.
In addition to policies, organisations must consider reputational risks as well as ethics.
Ethics
Privacy. Transparency. Unbiasedness. Explicability.
Privacy vs. Transparency
Data Privacy
“The right to the protection of personal data is not an absolute right; it must be considered in relation to its function in society and be balanced against other fundamental rights, in accordance with the principle of proportionality” (GDPR).
Once described by Supreme Court Justice Louis Brandeis as “the right to be let alone,” privacy is now best described as the ability to control data we cannot stop generating, giving rise to inferences we can’t predict.
Transparency
Leads to innovation and progress (e.g. the importance of data sharing in the search for covid vaccines)
Fosters competition (privacy vs. antitrust): data sharing reduces barriers to entry and enables entrepreneurship; proprietary data owner by a handful of firms -> power to large corporations
The public may have a right to aggregate information (if Google knows where all cars are, then maybe an individual should have the right to this knowledge as well.)
Internal transparency: enhances employee motivation
Compatible or Contradictory?
Some opinions:
Both privacy and transparency are tools of public good essential for the proper functioning of a democratic society, and both are defences against abuses of power. Yet there are inevitably times when they come into conflict. One must always be weighed against the other. (?)
Individual privacy and institutional transparency? Individuals should protect their personal information (identity). Institutions have an obligation to communicate pertinent information to their stakeholders and be transparent about what they do with personal information. Transparency and privacy go hand in hand. (?)
Lack of transparency in the big data value chain: individuals may not be sufficiently aware of what happens to their data after it is collected (sharing with third parties -> sub processor lists)
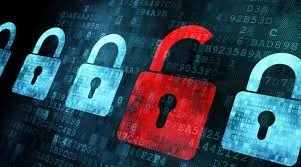
Privacy vs Cybersecurity
Cybersecurity
Cybersecurity: Health care, manufacturing, and financial services are the top three industries under attack, due to their personal data, intellectual property and physical inventory, and massive financial assets, respectively.
Cybersecurity is the protection of data from threat actors with motivations such as:
Cyberterrorists (cause harm, destruction)
State-sponsored actors (espionage)
Organized crime (money)
Cybercrime-as-a-service: hacker groups sell data
Hacktivists (ideological statements, exposing secrets or causing disruption to firms they deem evil)
Insiders:
The Biggest Cybersecurity Threats Are Inside Your Company (HBR, 2016)
60% of attacks are carried out by insiders (IBM 2016 Cyber Security Intelligence Index): 3/4 malicious intent & 1/'4 inadvertent actors
Human errors (misaddressed emails, confidential data sent to insecure home systems), leaked passwords (steal competitive information, sell data), stolen identities (often through malware or phishing; often hackers can then increase a user’s access leading them to more sensitive information)
Privacy and Cybersecurity are converging (HBR, 2019).
Privacy is moving from an immaterial, political concept to a tangible concern
Line between privacy and security teams is beginning to blurr (legal & privacy, technical)
In the past, the threat of unauthorized access to our data used to pose the biggest danger to our digital selves. Today, the biggest risk to privacy and security is the threat of (unintended) inferences, due to the power of increasingly widespread machine learning techniques. Examples include:
Threat to anonymity — like when a group of researchers used machine learning techniques to identify authorship of written text based simply on patterns in language.
Reveal health information — like when researchers used online search history to detect neurodegenerative disorders such as Alzheimer’s.
Unbiasedness. Explicability.
When decisions are based on algorithms (based on predictions made by AI/ML), the results should, ideally, be unbiased and the organization should be able to explain them. Both requirements are challenging.
Bias
Two sources of bias: 1. The training data 2. The algorithm
An AI system that makes automated loan decisions may be based on an unbiased algorithm but the overall result may be biased, due to the input data.
Examples:
In 2014, Amazon developed a recruiting tool for identifying software engineers it might want to hire; the system swiftly began discriminating against women, and the company abandoned it in 2017.
In 2016, ProPublica analyzed a commercially developed system that predicts the likelihood that criminals will re-offend, created to help judges make better sentencing decisions, and found that it was biased against blacks.
Bias against groups easier to address than fairness to individuals
Explicability
Explaining AI/ML systems and making them more transparent mitigates the harmful effects of bias.
Relates to accountability - who is responsible for accidents of self-driving cars?
The difficulty of explaining AI/ML systems is best illustrated with an exercise:
Use the inputs ICML (International Conference on Machine Learning); 2017; Australia; Kangaroo; Sunny.